Managing data quality in research: How not to get garbage data?
Tools to obtained and process a large amount of high-quality data are available. However, these tools along are not enough to obtain high quality data.
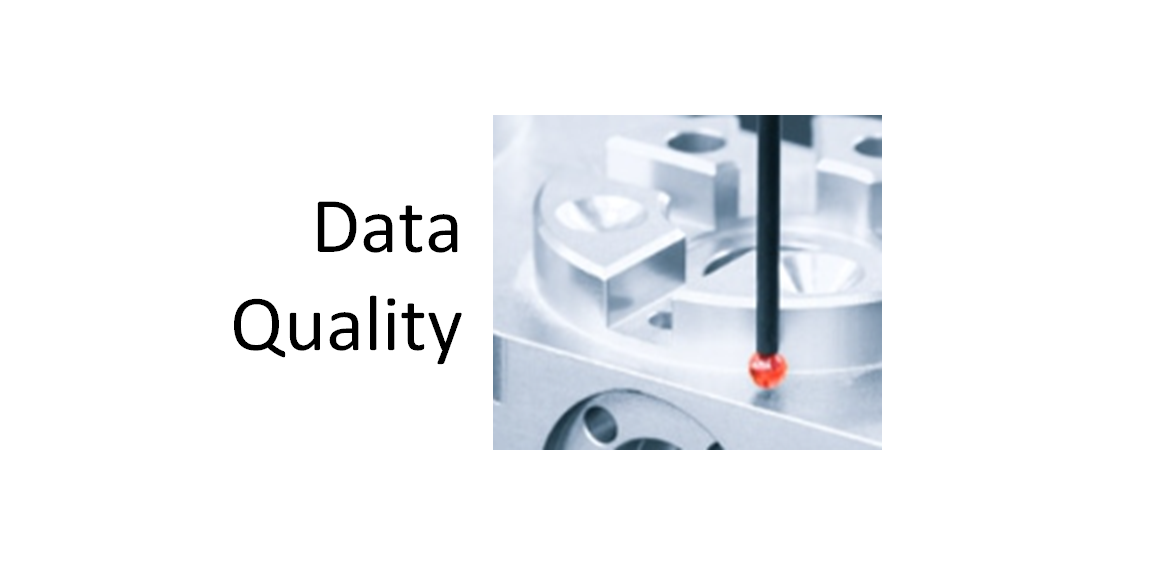
Data-driven research and development (R&D) activities have become current trends due to the advancement in algorithm, including machine learning and affordable computational tools (memory and processor).
Tools to obtained and process a large amount of high-quality data are available. However, these tools along are not enough to obtain high quality data.
It is important to remember bad or garbage data will destroy the whole R&D activities. Because these data will shape all aspects of the activities.
As in programming and data processing, we say “garbage in, garbage out”!
In this post, we will look at how we can avoid bad or garbage data so that we only get high-quality data to drive our R&D activities.
With high-quality data, we will get meaningful and impactful results from our R&D activities.
READ MORE: The role of measurement uncertainty in quality inspection
How to avoid collecting garbage data
Since data quality in data-driven R&D is important, we need to deliberately maintain data quality consistently.
Managing data quality in a large R&D team is challenging.
In this case, we take a case study in R&D in manufacturing and product development involving dimensional measurement processes to collect part geometry data.
Product developments and data analysis use results from the dimensional measurement of the parts geometries.
Things to consider on how to maintain measurement data quality consistency are as follows:
Instrument management
When we purchase a measuring instrument from a manufacturer. The purchase instrument will have a performance measure. The manufacturer provides this performance measure or metric.
In dimensional and geometrical metrology, a measuring instrument performance is specified by maximum permissible error (MPE) parameter.
For example, a coordinate measuring machine (CMM) will have a manufacturer defined MPE of $\pm (2 + \frac{L}{1000}) \mu m$ where $L$ is in mm.
This MPE means the instrument has a dimensional error of up to $2\mu m$ plus $1 \mu m$ for a measured length of $1m$.
The first thing we need to make sure our instruments work within their manufacturer specifications.
Hence, we need a performance verification process periodically applied to our instruments to make sure the instruments always work in their expected accuracy.
Measurement procedure
After we sure we periodically implement performance verification to our instruments, the next thing is to create a good and standardise measurement procedures.
Remember random procedure means random results!
We need to establish a set of procedure in measuring (including instrument set up and part placement as well as fixturing system) and processing (analysing) data.
We must make sure all operators are well trained on how to correctly use measuring instruments (including parameter settings and their effects).
In addition, the operator should also be well trained on the basics of statistics. They should understand the concept of randomness, statistical distributions and uncertainty estimations.
Calibration and measurement uncertainty
These aspects determined whether a measurement result is reliable or not!
Calibration, in a simple term, is that we compared our measurements with something that is more accurate and traceable to the definition of metre.
Commonly, calibration of a measuring instrument is performed by the instrument’s manufacturer in their workshop before sending the instrument to a customer.
For example, for CMM, calibration of determining the volumetric errors map of a CMM machine is conducted by the manufacturer of the CMM.
Next, we must always provide the estimation of uncertainty for our measurement results. only by measurement uncertainty, we can reliably compare measurement results of the same quantity from different measurement processes, with for example, different operators, time and measurement parameters.
Measurement uncertainty can be estimated by using GUM method, spreadsheet method or Monte-Carlo method.
Work manner
Work manner is important! This manner is a non-technical aspect but maybe the most important one.
All members should have good manner in working together as a team. This manner is a lubricant to reduce friction when team members interact among team members with different background, culture and expertise.
Manner will foster teamwork and cause a team to thrive moving toward the team’s R&D visions.
Only with manner, a R&D team can function properly! Team member should respect each other work and results and carefully processes their data for analysis as well as each other measurement setups.
This manner needs to be deliberately conveyed by the team leader.
Some tips to raise manner are by providing team building activities, personal discussions and brainstorming or join a professional team building training.
Work culture
Still related to manner and also a non-technical aspect, a good manner will create a good work culture.
High quality data can only be obtained with a good work culture.
Work culture includes respecting all established procedures to perform measurements even though the procedures require a long time to follow.
The procedures include carefully place and locate the part with a correct fixturing setup, gently place the part and tighten the fixture, cleaning the part surface before measurement.
An incorrect part placement and fixturing can introduce error up to few hundred micrometres. Meanwhile, dust on surfaces may cause measurement error of few micrometre (due to the dust attached to the tip of a stylus probe and cause wrong part surface touch triggering).
We need to follow the established measurement procedures meticulously by heart. That is why patient is key to obtained high quality measurement data.
READ MORE: Research how to: A practical guide
Conclusion
In this post, we have discussed about managing data so that we don’t get garbage data. When we get garbage data, our R&D activities will also produce garbage or bad results.
We discussed five aspects on how not to get garbage data in a case of a product R&D activity in manufacturing where we need to collect dimensional data of part geometries.
The five aspects we have discussed include measuring instrument management, measurement procedure, measurement uncertainty and manner and work culture.
These five aspects if we can manage well, we can get high quality data leading to high quality R&D results.
You may find some interesting items by shopping here.
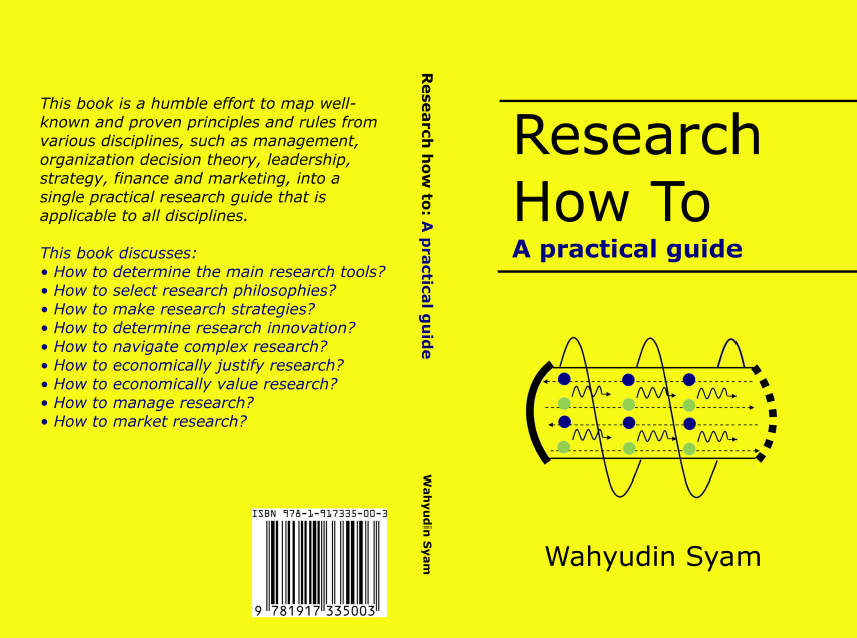